In today’s fast-changing business world, using big data and business intelligence is key. This guide explores big data business intelligence. It shows how to use data analytics for better decision-making and growth in 2025.
As data grows, so does its value. Businesses see the power of business intelligence and big data. They use it to find insights, improve operations, and serve customers better. This guide will help you understand and use big data business intelligence to make smart choices for your company.
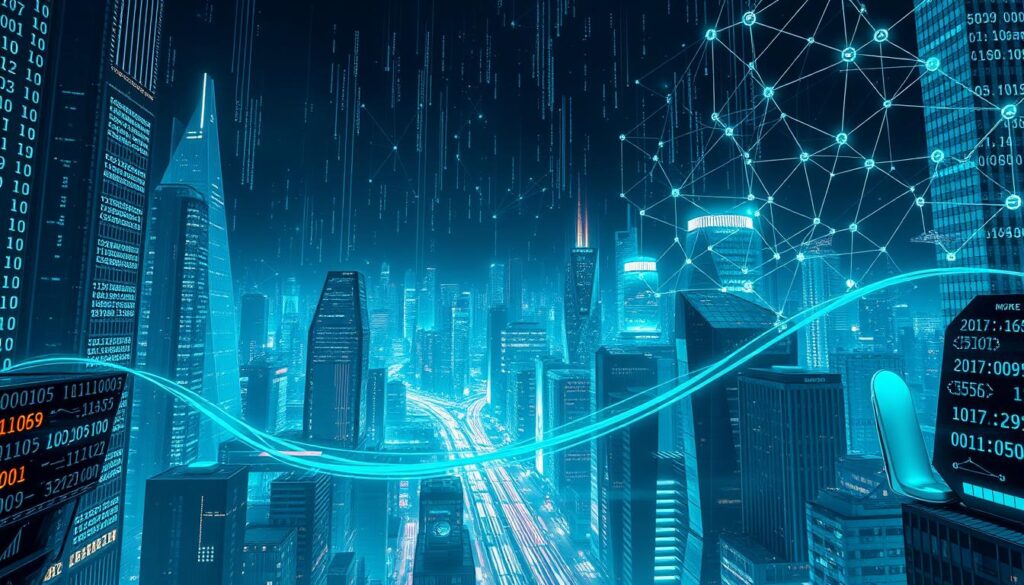
This guide is for everyone, from experienced leaders to newcomers. It covers the basics and the latest in big data business intelligence. You’ll learn about modern BI, data analytics, and how big data affects business. It’s your guide to becoming a data-driven leader.
Understanding Big Data Business Intelligence
In today’s fast-paced business world, using data wisely is key. Big data business intelligence, or big data BI, is a way to use lots of data and smart analysis to make better choices. It helps businesses make decisions based on solid data.
Defining Modern Business Intelligence
Business intelligence (BI) turns raw data into useful insights for making big decisions. But, old BI methods are outdated. Big data BI tackles the big challenge of handling huge, complex data sets that old systems couldn’t manage.
The Evolution of Data Analytics
Data analytics has changed a lot lately. Old BI dealt mainly with data from inside the company. But big data analytics looks at all kinds of data, like social media and sensor data. This lets businesses understand more about themselves, their customers, and the market.
Core Components of Big Data BI
- Data collection and integration: Gathering and combining data from many sources, both structured and unstructured.
- Big data storage and management: Using solutions that grow with data to keep costs down.
- Advanced analytics and modeling: Using complex stats and machine learning to find new insights.
- Real-time data processing and visualization: Giving quick access to insights with interactive dashboards.
By using big data BI, companies can stay ahead, improve operations, and better serve customers. They can also make smarter, more informed decisions.
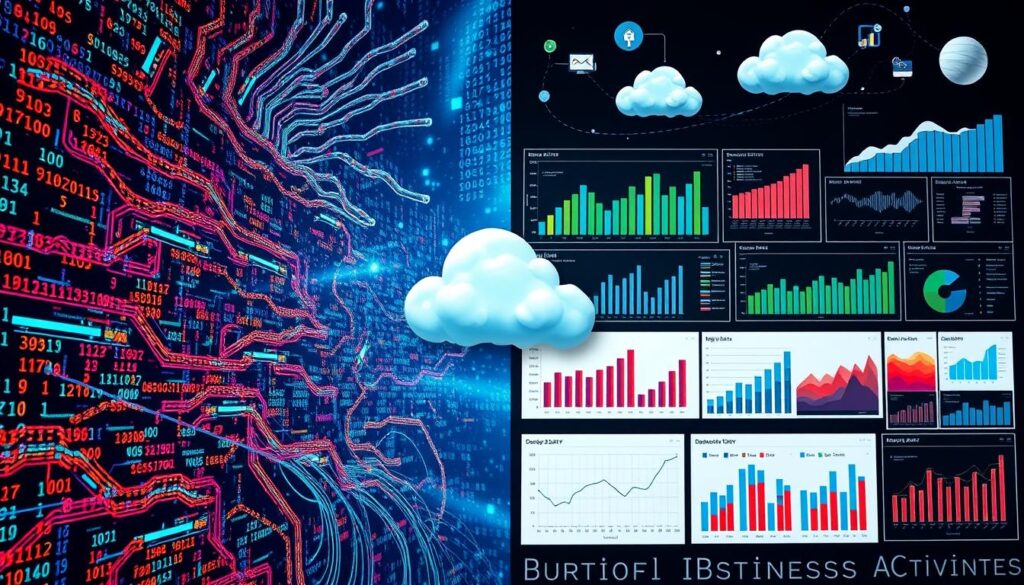
The Impact of Big Data on Modern Business Operations
In today’s digital world, big data analytics is changing how businesses work. By leveraging big data for business intelligence, companies get deep insights into their customers and operations. This business intelligence and analytics from big data to big impact is seen in many industries.
The retail sector is a great example of big data analytics examples. Top retailers use big data to improve their supply chains and personalize customer experiences. They also make smart pricing decisions. This has made their operations more efficient, customers happier, and profits higher.
In the financial world, big data helps spot fraud, assess risks, and find new investment chances. By digging into lots of customer data, banks and financial firms can offer better products. This leads to happier customers and more loyalty.
The healthcare field has also seen big changes thanks to big data BI solutions. Hospitals and research centers use big data to predict diseases, manage resources, and create custom treatment plans. This has improved patient care and made healthcare more efficient.
In all these fields, the effect of big data analytics examples is clear. By leveraging big data for business intelligence, companies can make better choices, excel in operations, and stay competitive in a fast-changing market.
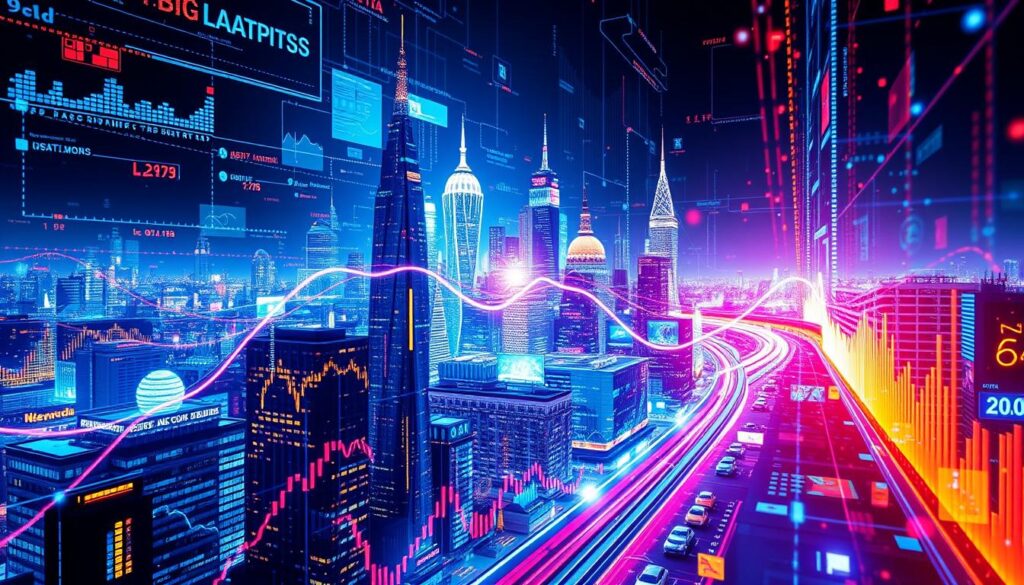
Key Differences Between Traditional BI and Big Data Analytics
The business world is changing fast. This change makes the difference between traditional business intelligence (BI) and big data analytics clear. Knowing these differences is key for companies wanting to use data well.
Processing Capabilities Comparison
Traditional BI uses data from inside the company, processed in batches. Big data analytics, however, works with lots of different data from inside and outside the company. It uses tools like Hadoop and Spark to handle data quickly.
Data Storage Solutions
Traditional BI uses relational databases to store data. Big data analytics, though, uses many different storage options. These include NoSQL databases, data lakes, and cloud storage. These options are better for handling big, varied data.
Analysis Methods and Approaches
- Traditional BI looks at past data to find ways to improve. It uses set reports and queries for this.
- Big data analytics, though, looks to the future. It uses advanced models and AI to predict trends and suggest actions.
Understanding the differences in how traditional BI and big data analytics work helps companies make better choices. This choice can lead to smarter, more effective business decisions.
Essential Big Data Analytics Tools and Platforms
In today’s big data world, businesses need many analytics tools and platforms. They range from data warehousing solutions to business intelligence software and advanced data visualization tools. Knowing what these tools can do helps you pick the best ones for your organization.
Comprehensive Data Warehousing Solutions
Platforms like Amazon Redshift, Google BigQuery, and Microsoft Azure Synapse Analytics are key. They help store and manage lots of data. These solutions offer scalable storage and advanced querying, making it easy to integrate with other tools.
Powerful Business Intelligence Software
Top business intelligence platforms, like Tableau, Power BI, and Qlik, turn data into insights and dashboards. They let users explore data, create reports, and find trends. This helps make strategic decisions.
Cutting-Edge Data Visualization Tools
Tools like Matplotlib, Plotly, and D3.js make complex data easy to understand. They create graphics and interactive visualizations. This helps share insights, start discussions, and align the organization.
Tool | Key Features | Use Cases |
---|---|---|
Amazon Redshift | – Scalable data warehousing – High-performance SQL queries – Integration with other AWS services | – Enterprise-level data analytics – Predictive modeling – Real-time decision support |
Tableau | – Intuitive data visualization – Interactive dashboards – Self-service data exploration | – Exploratory data analysis – Reporting and KPI tracking – Collaborative data-driven insights |
Matplotlib | – Extensive library of 2D and 3D plots – Customizable visualizations – Integration with Python ecosystem | – Data exploration and prototyping – Scientific and academic research – Embedded visualizations in applications |
Using the right big data analytics tools and platforms unlocks your data’s full potential. This leads to better decision-making at all levels.
Implementing Business Intelligence Solutions
Modern businesses need to adopt strong business intelligence (BI) solutions to use big data effectively. A successful implementation requires a strategic approach. This includes setting up the right infrastructure and building a dedicated team.
Strategy Development
Creating a solid BI strategy is key. It starts with understanding your data needs and aligning BI goals with your business objectives. You also need a plan for how to deploy it. Think about your data sources, what you can analyze, and how it will work with your current systems.
Infrastructure Requirements
You need a strong, flexible infrastructure for BI. This might include cloud-based platforms, data warehouses, and secure storage. It’s important to have smooth data flow, fast processing, and reliable reports. This way, you can get valuable insights from your data.
Team Structure and Roles
- Build a BI team with skills in data management, analytics, and business operations.
- Make sure everyone knows their role, like data engineers, business analysts, and BI consultants.
- Keep communication open and teamwork strong to create a data-driven culture.
Key Roles | Responsibilities |
---|---|
Data Engineer | Designing and maintaining data infrastructure, ensuring data quality and security |
Business Analyst | Translating business requirements into data-driven insights and actionable recommendations |
BI Consultant | Providing strategic guidance, implementing BI solutions, and optimizing performance |
By focusing on these important steps, businesses can make the most of their BI solutions. This leads to better decision-making throughout the company.
Data Visualization Techniques and Best Practices
In the world of big data, turning complex info into useful insights is key. Using big data visualization tools and smart techniques helps businesses share insights clearly. This leads to better decision-making and tapping into data’s full potential.
Keeping it simple is a main rule of good data visualization. Don’t overwhelm people with too many charts. Instead, focus on the most important info in a clear, easy-to-understand way. Use colors, interactive dashboards, or storytelling to guide your audience.
Choosing the right type of visualization is also crucial. Depending on your data and goals, pick from scatter plots, bar charts, line graphs, or pie charts. The goal is to find the best match for your data and message.
Details matter too. Good data labeling, axis scaling, and design make your visuals clear and impactful. By making your visualizations appealing and easy to understand, you can turn data into actionable insights. This helps drive important business decisions.
Best Practices for Effective Data Visualization
- Keep it simple: Avoid cluttered visualizations and focus on the essential information.
- Choose the right visualization type: Match the chart or graph to the specific data and insights you want to convey.
- Prioritize clarity and legibility: Use clear labeling, appropriate axis scaling, and a clean design.
- Utilize color strategically: Use color to highlight important data points and create visual hierarchy.
- Encourage interactivity: Incorporate dynamic elements that allow users to explore the data further.
- Tell a story: Use data visualization techniques to guide your audience through the insights and their implications.
Visualization Type | Best Use Case | Advantages |
---|---|---|
Line Chart | Tracking trends over time | Effective for visualizing continuous data and identifying patterns |
Bar Chart | Comparing categorical data | Straightforward and easy to interpret, suitable for side-by-side comparisons |
Scatter Plot | Identifying relationships and correlations between variables | Useful for exploring the relationship between two or more data points |
Pie Chart | Displaying part-to-whole relationships | Visually appealing and intuitive for showing the composition of a whole |
Real-time Analytics and Decision Making
In today’s fast-paced business world, real-time data analytics tools are key. They help companies make quick, informed decisions. This keeps them ahead of the competition.
Stream Processing Technologies
Stream processing technologies like Apache Kafka and Amazon Kinesis are vital. They let businesses analyze data as it comes in. This way, companies can quickly respond to market changes.
Real-time Monitoring Systems
Real-time monitoring systems are essential for business intelligence today. They track important performance indicators and spot trends and anomalies fast. This lets leaders act quickly to solve problems.
Instant Decision Support
Real-time data analytics tools help businesses make fast, informed decisions. They analyze data as it happens. This gives leaders the info they need to act quickly and stay competitive.
Feature | Traditional BI | Real-time Analytics |
---|---|---|
Data Processing | Batch processing | Stream processing |
Decision-making | Delayed, reactive | Instant, proactive |
Insights | Historical, static | Dynamic, actionable |
Responsiveness | Slow, limited | Rapid, adaptive |
By using real-time analytics, businesses can stay ahead. They can quickly adapt to market changes. This leads to better, data-driven decisions that drive success.
Cloud-Based Business Intelligence Solutions
In today’s big data world, cloud-based business intelligence (BI) solutions are changing the game. They help organizations make data-driven decisions. These platforms offer many benefits that can transform how businesses use big data analytics.
One big plus of cloud-based BI is its scalability. Businesses can grow their BI capabilities as their data grows. They don’t need to spend a lot on hardware. This makes it easy to keep up with market changes and business needs.
Cloud-based BI also makes insights more accessible. Users can get to important business insights from anywhere, at any time. This helps teams work better together. It creates a culture that uses data to make strategic decisions.
Feature | Cloud-Based BI | Traditional BI |
---|---|---|
Scalability | Highly scalable | Limited scalability |
Accessibility | Anytime, anywhere access | Restricted to on-premises access |
Cost-Effectiveness | Reduced upfront costs, pay-as-you-go model | Significant upfront investment in hardware and software |
Maintenance and Updates | Automatic updates and maintenance by the cloud provider | Requires internal IT resources for maintenance and upgrades |
When looking at cloud-based BI, businesses should think about data security and compliance. They should also consider how well the platform fits with their current systems. By choosing the right cloud-based BI, organizations can make the most of big data. This gives them an edge in today’s data-driven world.
Data Security and Compliance in Big Data BI
Businesses are using big data and business intelligence more and more. This means they need to focus on keeping data safe and following rules. It’s key to protect sensitive data to stay ahead in the market.
Regulatory Requirements
Companies in fields like healthcare, finance, and government face many rules. They must follow laws like HIPAA, GDPR, and CCPA to avoid big fines and damage to their reputation. Keeping up with these rules and having strong data management is vital.
Security Protocols
Strong security is the base of a safe big data BI system. This includes using advanced encryption, controlling who can access data, and having systems to find and fix threats. By tackling these issues, companies can keep their data safe and their edge in the market.
Privacy Measures
Keeping personal data safe is a big part of following big data BI rules. Companies must make sure they collect, store, and use data the right way. This means getting clear consent, making sure data is anonymous, and being open about how data is used. This builds trust with customers and others.
By focusing on data security and following the rules, companies can use big data and business intelligence well. This helps them keep their data safe and maintain trust, which is crucial for success over time.
Predictive Analytics and Machine Learning Integration
In the world of big data business intelligence, predictive analytics and machine learning are changing the game. These technologies help businesses use their data better. They can forecast trends, spot patterns, and make smarter choices.
Predictive analytics uses past data and models to guess what will happen next. It helps businesses see market changes, customer actions, and risks coming. This way, they can make decisions that grow their business and run things more smoothly.
Machine learning lets systems learn and get better on their own. It looks at lots of data to find complex links and hidden insights. When predictive analytics and machine learning work together, businesses can really use their data to grow.
This team-up helps businesses predict better, run things more smoothly, and make smarter choices. They can guess when customers might leave, improve marketing, find fraud, and make supply chains better. The possibilities are endless.
By using predictive analytics and big data analytics, businesses can thrive in a changing world. This smart use of technology helps them make better decisions, stay ahead, and grow for the long haul.
Industry-Specific Applications and Use Cases
In today’s world, businesses in many fields use advanced analytics to stay ahead. This includes healthcare, financial services, and retail. Data insights are changing how companies work and serve their customers.
Healthcare Analytics
In healthcare, healthcare business intelligence is changing patient care and making operations smoother. It uses data from electronic health records and more. This helps in making better decisions, using resources wisely, and spotting disease outbreaks sooner.
Financial Services Analytics
The financial world has always been big on data analytics. Financial services analytics is key for managing risks, catching fraud, and offering better customer service. Banks and financial firms use data to spot trends, find fraud, and customize services for their clients.
Retail Intelligence
In retail, retail data analytics helps understand shoppers, manage stock, and boost sales. Retailers use data from online and in-store sales, social media, and feedback. This helps them offer a better shopping experience, guess demand, and make decisions that help their bottom line and keep customers coming back.
In these different fields, using big data smartly opens up new chances for growth and improvement. As companies keep using data, the possibilities for big changes in all areas are endless.
ROI Measurement and Performance Metrics
Businesses invest a lot in big data and business intelligence (BI). It’s key to measure the return on investment (ROI) and track important metrics. This guide will help you see if your BI efforts are paying off and if your data analytics investments are worth it.
Measuring ROI for Big Data BI
Figuring out the ROI of big data BI projects is tricky. The benefits aren’t just about money. To measure ROI, look at:
- Cost savings from better operations
- More revenue from smart decisions
- Better customer satisfaction and loyalty
- Improved risk management and following rules
- Advantages over competitors from data insights
Key Performance Metrics for Big Data BI
Tracking ROI is just part of the story. You also need to watch other performance metrics. Key ones include:
- Data quality and accuracy
- How fast data is processed and analyzed
- How much users engage with BI tools
- The impact of insights on decision-making
- Cost savings from better operations
- Revenue growth from smart business moves
Metric | Description | Importance |
---|---|---|
Data Quality | Checks if the data is accurate, complete, and up-to-date | Ensures the insights from data are trustworthy |
Analysis Speed | Measures how quickly data is analyzed for fast decisions | Helps respond quickly to market changes and customer needs |
User Adoption | Looks at how much employees use and engage with BI tools | Shows if the BI solution meets user needs and drives change |
By watching these ROI and performance metrics, businesses can see if their big data BI efforts are working. This helps make smart choices about future investments and priorities.
Future Trends in Big Data Business Intelligence
The world of big data and business intelligence is always changing. The big data and business intelligence msc and msc business analytics greenwich university programs are leading the way. They teach students the skills needed for this fast-paced field.
Artificial Intelligence (AI) and Machine Learning (ML) are becoming key in business intelligence. These technologies will help with better predictive analytics and quicker decision-making. The big data and business intelligence msc university of greenwich is already teaching these important topics.
Edge computing is also set to grow in big data business intelligence. It allows for faster analytics by processing data closer to its source. This change will help businesses make decisions in real-time, changing how they use data.
- Advancements in Artificial Intelligence and Machine Learning
- The rise of Edge Computing for faster, more responsive analytics
- Increased integration of Augmented Reality and Virtual Reality for data visualization
- Expansion of cloud-based BI solutions to drive accessibility and scalability
- Growing emphasis on data ethics and responsible data management
The big data and business intelligence msc, msc business analytics greenwich university, and big data and business intelligence msc university of greenwich programs are key to the future. By keeping up with these trends, students will be ready to lead in the business intelligence world.
Common Implementation Challenges and Solutions
Starting big data business intelligence (BI) can face many challenges. But, with smart strategies, these problems can be solved. Issues range from technical to organizational and resource-related. Let’s look at how to tackle these common hurdles and make sure your big data BI projects succeed.
Technical Hurdles
One big technical challenge is combining different data sources. It’s also hard to manage the huge amounts, types, and speeds of big data. To solve this, invest in strong data integration and management tools like Apache Spark or Hadoop. They can handle big data’s complexities.
Also, using a flexible, scalable, and cloud-based BI setup helps. It overcomes hardware and storage limits.
Organizational Barriers
Resistance to change and a lack of data culture can slow down big data BI adoption. To get past these, it’s key to win over stakeholders and leaders. Show them how big data BI can give your business an edge.
Offering thorough training and change management programs is also vital. It helps your team adjust to making decisions based on data.
Resource Constraints
Big data BI projects need a lot of talent, tech, and infrastructure. To deal with these costs, consider teaming up with seasoned big data analytics providers. Or, use cloud-based BI solutions for cheaper access to top tech and skills.
Also, training your current team can build the skills needed for big data BI. This way, you can support your projects without needing to hire more people.
FAQ
What is the relationship between big data and business intelligence?
Big data and business intelligence (BI) are closely linked. Big data refers to large, complex datasets that businesses analyze for insights. Business intelligence uses technologies and strategies to turn data into useful information for making decisions. By combining big data analytics with BI, organizations can use all their data to make better, more informed decisions.
How does big data differ from traditional business intelligence?
Big data and traditional BI differ in several ways. Big data can handle more data, faster, and in different formats than traditional BI. Big data also uses advanced techniques like predictive modeling and machine learning. Traditional BI focuses more on looking back at data and reporting.
What are the essential tools and platforms for big data business intelligence?
Key tools for big data BI include data warehousing solutions like Hadoop and Spark. Also, business intelligence software like Tableau and Power BI, and data visualization tools like D3.js and Plotly are essential. These tools help organizations store, process, analyze, and share insights from large datasets.
How can businesses implement successful big data BI solutions?
To implement successful big data BI, a clear strategy is needed. Ensure the right infrastructure and technology are in place. Also, build a team with the right skills and roles. Focus on effective data visualization and real-time analytics to make data-driven decisions.
What are the industry-specific applications of big data business intelligence?
Big data BI has many uses across industries. In healthcare, it helps improve patient care and disease prevention. In finance, it aids in fraud detection and risk management. In retail, it helps with demand forecasting and supply chain optimization.
What are the future trends in big data business intelligence?
Future trends in big data BI include more predictive analytics and machine learning. Cloud-based BI solutions are also on the rise. Real-time data processing and decision support are becoming more important. Advancements in edge computing and IoT will further enhance big data BI capabilities.